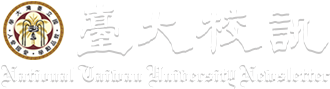
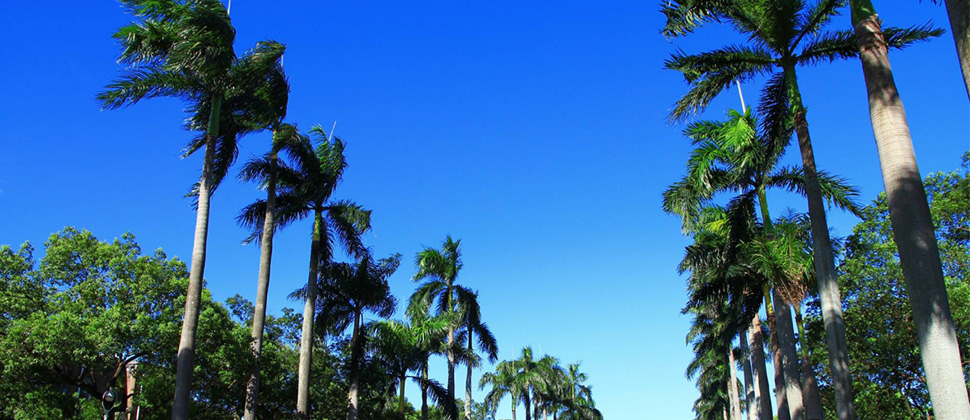
6/17 分子生醫影像研究中心講座:大腦結構運算分析
大腦結構的運算分析主要利用T1權重影像與擴散權重影像來分別對宏觀與微觀結構進行探討。本此演講主題著重在此兩種結構性影像的分析演算法介紹及其可能應用。
Computational anatomy (CA) is an emergent field studying the anatomical characteristics of human brain. Among imaging modalities of magnetic resonance imaging (MRI), T1-weighted images (T1W) and diffusion weighted images (DW) are usually employed in CA to investigate the macroscopic and microscopic structural characteristics, respectively. Image registration is one of the most important tasks in CA, where the primary goal of image registration is to accommodate the images of a group of subjects to a common coordinate system. By formulating the problem of image registration in terms of diffeomorphism group, the large deformation diffeomorphism metric mapping (LDDMM) algorithm is capable of estimating the geodesic (i.e., shortest path) between images. We developed a registration method for diffusion spectrum imaging (DSI) datasets under the framework of LDDMM. This method, LDDMM-DSI, made use of the fact that a DSI dataset is 6D, and generalized the original 2D/3D LDDMM algorithm to the 6D case with some modifications made for the DSI datasets. In this manner, the conventional reorientation problem that arises from transforming DW datasets was avoided by making the DSI datasets capable of being freely deformed in the q-space, leading LDDMM-DSI capable of achieving nicely registration between DSI datasets. To facilitate group analyses of DW datasets, we constructed a DSI template in the ICBM-152 space from 122 healthy adults. This high quality template, NTU-DSI-122, was built through incorporating the macroscopic anatomical information using high-resolution T1W images and the microscopic structural information obtained from DSI datasets. NTU-DSI-122 matched to the ICBM-152 space very well across the whole brain, including the deep white matter (WM) regions and the superficial WM regions, therefore, it can serve as a representative DSI dataset for a healthy adult population. To address regression problems where the data sit on Riemannian manifolds, we proposed the multiple geodesic regression (MGR) method. The MGR method modifies the geodesic regression (GR) framework; while GR considers a single scalar explanatory variable, MGR allows multiple scalar explanatory variables. The MGR method was applied to a diffeomorphism group, along with the LDDMM framework, to study the aging effects on the morphological variations of human brain across adult lifespan. The results showed that the aging factors explained significant (p=0.0001) linear and/or quadratic morphological variations of the brain, consistent with the known aging patterns. This real application reveals that MGR is potentially useful in studying brain morphological changes in association with genotypic or phenotypic features of interest. As MGR is developed for general Riemannian manifolds, further scientific research and clinical applications can be conducted to explore its potential values.
講題: 大腦結構運算分析 Computational anatomy for human brain
講者:徐泳欽博士
時間:6/17(五)11:30
地點:徐泳欽博士國立臺灣大學校總區生物科技館R101